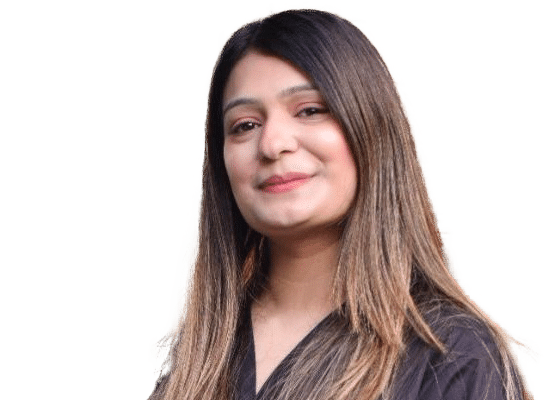
Data & AI Evangelist
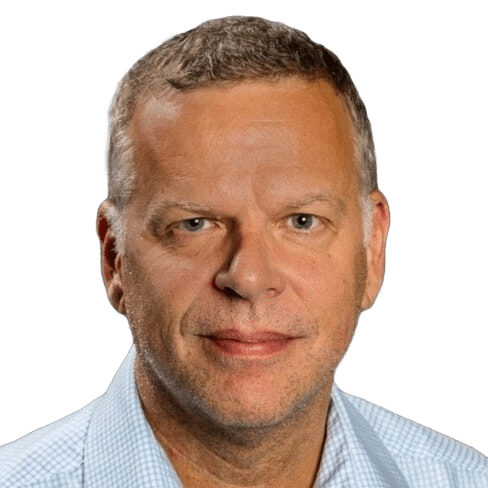
Principal Data Architect
Subscribe to the newsletter
The fast adoption of generative AI across various industries has opened scores of opportunities for organizations to transform their operations. Over the past few years, AI development has been limited to professional data scientists who developed highly trained AI models. Today, with innovative generative AI models like OpenAI’s ChatGPT, Google Bard, GitHub Copilot, and Meta LIama, millions of users are now accessing powerful AI models to automate tasks with unprecedented speed and innovation.
Salesforce reported that almost 86% of IT leaders acknowledge that generative AI will have a massive impact on organizations in the future. Owing to generative AI’s capabilities in driving insights and decision-making, a recent McKinsey survey found that nearly half (40%) of organizations plan to boost their overall investment in AI.
This rapid pace of generative AI adoption forces us to confront the long-term implications. Are we ready to trust these tools and manage their impact responsibly? The answer lies in our commitment to risk management and ethical data governance, which will shape the future of generative AI and define our ethical stance as organizations.
Enterprise data generated from siloed sources, and the absence of a data integration strategy, creates challenges in provisioning data for generative AI applications. An end-to-end strategy for data governance and management is required–ranging from ingesting, storing, and querying data to analyzing, visualizing, and running AI and ML models.
This blog post explores the importance of data governance in the age of Generative AI, addressing generative AI governance challenges and building a strategy to achieve trust and transparency and building a strategy to achieve trust and transparency.
Data governance for a thriving generative AI ecosystem
The skyrocketing adoption of AI in recent years has yielded incredible benefits, but it also requires vast amount of data to train these AI systems. This vast amount of data raises concerns regarding data lineage, trust, and privacy, raising the need for data governance services to uncover the true potential of generative AI. Effective data governance mitigates these concerns and empowers organizations by ensuring the quality, visibility, and reliability of data used to fuel their data and AI operations.
Instead of limiting the use of generative AI models for sensitive data, organizations should prioritize establishing governance to make secure choices and boost productivity. On top of that, robust data governance is also essential for effective risk management and regulatory compliance. Without it, organizations are at a heightened risk of AI-related data leaks, data breaches, and biased outputs.
Further readings: Explore data governance as a strategic asset for business growth.
Exploring the need for strong AI data governance in Generative AI? Challenges explained
While investment in generative AI offers incredible value to organizations, it also comes with a unique set of challenges that organizations face. Therefore, data governance for Generative AI is essential to ensure that the data used for training models is accurate, unbiased, complies with privacy regulations, and is securely managed. It helps mitigate risks associated with data misuse, promotes transparency in decision-making processes, and enhances operational efficiency by streamlining data management practices.
Let’s explore some of these challenges in detail:
Lack of guidelines
Generative AI is still in its early stages of development, which means there is currently a lack of established AI governance frameworks. Without clear data governance strategies, organizations struggle to define standardized practices for managing data quality, privacy, and security. This includes addressing ethical considerations specific to AI applications. This lack of guidelines complicates decision-making processes regarding data acquisition, processing, and usage. It leads to uncertainties in compliance with regulatory requirements and potential risks, such as biases in AI models.
Lack of visibility
The lack of visibility is a critical challenge in data governance for Generative AI. It refers to the difficulty in tracking and understanding how data is sourced, processed, and used within AI models, particularly those that generate content autonomously. Generative AI’s complexity, driven by complex algorithms and vast datasets, poses challenges in maintaining transparency in data flows and transformations. Without clear visibility, organizations face difficulties in identifying potential biases, errors, or ethical issues embedded in AI-generated outputs.
Data security
In the generative AI era, where data is integral to organizations, sharing sensitive information with AI models carries inherent risks. Data leaks and compliance issues can have severe consequences for organizations. To overcome this challenge, organizations must implement data security measures to protect their data while interacting with AI models. This includes encrypting sensitive data, controlling access, and implementing rigorous monitoring to mitigate the risks associated with AI integration.
Ethical considerations
The transformative potential of generative AI is accompanied by a significant challenge: bias. When AI models are trained on biased datasets, they can reinforce these biases in their outputs, leading to ethical concerns and potentially discriminatory outcomes. Addressing the challenge of Generative AI ethics is crucial for upholding ethical standards. However, the intricate nature of generative AI models complicates the identification and mitigation of bias, requiring robust strategies and continuous vigilance to ensure fairness and equity in AI applications.
Now, what can a good generative AI governance strategy do here?
A robust generative AI data governance strategy can effectively mitigate biases, ensure transparency in AI operations, and uphold ethical standards. By implementing rigorous bias detection mechanisms, and fostering accountability, such a strategy promotes the responsible use of AI to enhance the trust among users.
With a robust generative AI governance strategy, an organization can achieve several key objectives, such as:
- Ensure ethical use of AI data governance to prevent unethical practices.
- Identify and address potential risks associated with generative AI.
- Ensure compliance with relevant laws, regulations, and standards.
- Promote transparency in the decision-making processes of generative AI systems.
- Build trust among stakeholders in the use of generative AI technologies.
While commendable efforts are being made to embrace data governance in generative AI, a Gartner report stated that one-fifth of the organizations have established a generative AI governance strategy, while 42% are currently working on developing a strategy. This suggests that there is growing recognition of the importance of governance in managing the risks associated with generative AI.
How can you build your generative AI governance strategy?
Since a generative AI governance framework and strategy focuses on managing the generative AI model’s data, the goal is to ensure that this data is high-quality and unbiased. Additionally, it aims to guarantee that the data is secure and private, and used ethically and responsibly. By having a data governance strategy in place, organizations can leverage the power of generative AI while mitigating the risks. We’ve outlined a step-by-step approach to build your AI governance strategy for responsible generative AI use in data governance.
Step 1: Define your governance focus
Creating an Artificial Intelligence data governance strategy begins with a clear focus. The first step involves understanding your organization’s involvement with generative AI. This initial assessment sets the stage for the governance approach tailored for your organizations needs. The approach varies depending on whether you develop or enhance generative AI in-house or use third-party gen AI tools.
Suppose your organization chooses to build its generative AI model. In this case, the goal is designing a secure, robust AI governance software solution. This includes choosing training data, incorporating privacy in design, strategies to mitigate bias, and designing an effective user interface.
Step 2: Identify your AI landscape
After understanding your generative AI focus, map out all the generative AI systems currently in use within your organization. This will help you identify potential risks and overlaps across different departments. While mapping your AI systems, identify all generative AI systems, document their purpose, and assess their level of integration.
Creating a detailed AI landscape map gives you a clear picture of your organization’s current generative AI usage. This process will be beneficial for developing a governance framework that effectively addresses the specific needs of your AI environment.
Step 3: Build your governance framework
The third step focuses on establishing a robust AI data governance framework for your generative AI systems. This framework outlines the rules, and processes, that ensure the responsible and ethical use of generative AI within your organization.
Involve a diverse group of stakeholders to gain a well-rounded perspective on the potential implications of your generative AI systems. Moreover, organizations can also leverage existing governance frameworks as a foundation for their generative AI governance strategy. Evaluate your existing governance framework to identify and adapt areas to address the specific needs and risks of generative AI.
A new era for generative AI and data governance
In the past few years, data governance services have evolved beyond just risk and compliance. It now plays a crucial role in enabling advanced analytics, AI initiatives, and empowering users with self-service data access (data democratization). The explosive growth of AI and generative AI adoption necessitates a more agile and flexible approach to data governance. This field is poised for a significant evolution, driven by several key factors.
- Ensuring data quality and privacy for AI models is crucial.
- Data governance must facilitate responsible and ethical AI development.
- New AI-specific risks like fairness and transparency require tailored governance strategies.
- Compliance with emerging AI regulations will be an important consideration.
While there are many perspectives on how quickly and in what ways generative AI will be adopted, it is widely acknowledged that initiatives require strong data governance to ensure the responsible use of data. Organizations will find it challenging to create an AI strategy independently of their data strategy. Therefore, data governance must evolve to support this integrated framework as organizations move towards integrated data and AI strategies.
Build scalable AI data governance strategy with Confiz
Gaining and maintaining trust in generative AI systems depends on our collective commitment to robust data governance. Looking to the future, organizations embracing data governance practices in the age of generative AI will be well-positioned to maximize the technology’s transformative potential. This proactive approach will foster trust, mitigate risks, and pave the way for a future where generative AI flourishes for the benefit of all.
To discover how data governance in the age of generative AI can empower your organization, reach out to our experts. Email us at marketing@confiz.com to establish a data governance strategy that aligns with your business needs.